2018 CSDMS meeting-012
Log in (or create account for non-CSDMS members)
Forgot username? Search or email:CSDMSweb@colorado.edu
"4) EarthLab" is not in the list (1) Downscaling climate models for regional applications, 2) Dakota, 3) ANUGA, 4) Artificial Intelligence & Machine Learning, 5) Will not attend a clinic) of allowed values for the "CSDMS meeting select clinics3 2018" property.
Browse abstracts
Towards a Quantitative Understanding of Parameters Driving Submarine Slope Failure: A Data Mining and Machine Learning Approach
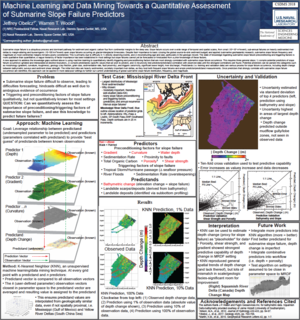
Submarine slope failure is a ubiquitous process and dominant pathway for sediment and organic carbon flux from continental margins to the deep sea. Slope failure occurs over a wide range of temporal and spatial scales, from small (10e4-10e5 m3/event), sub-annual failures on heavily sedimented river deltas to margin-altering and tsunamigenic (10-100 km3/event) open slope failures occurring on glacial-interglacial timescales. Despite their importance to basic (closing the global source-to-sink sediment budget) and applied (submarine geohazards) research, submarine slope failure frequency and magnitude on most continental margins remains poorly constrained. This is primarily due to difficulty in 1) directly observing events, and 2) reconstructing age and size, particularly in the geologic record. The state of knowledge regarding submarine slope failure preconditioning and triggering factors is more qualitative than quantitative; a vague hierarchy of factor importance has been established in most settings but slope failures cannot yet be forecasted or hindcasted from a priori knowledge of these factors.
A new approach to address the knowledge gaps outlined above is using machine learning to quantitatively identify triggering and preconditioning factors that are most strongly correlated with submarine slope failure occurrence. This occurs in three general steps: 1) compile potential predictors of slope failure occurrence gridded and interpolated at desired resolution, 2) compile predictands (specific values that we wish to predict), and 3) recursively test predictor/predictand correlation with observed data until the strongest correlations are found. Potential predictors can be parsed into categories such as morphology (gradient, curvature, roughness), geology (clay fraction, grain size, sedimentation rate, fault proximity), and triggers (seismicity, significant wave height, river discharge). Predictands (i.e. training data) are various proxies for slope failure occurrence, including depth change between bathymetric surveys and sediment shear strength. The initial test sites are heavily sedimented, societally important river deltas, as they host both frequent slope failures and ample predictor/predictand measurements. Once predictors that strongly correlate with submarine slope failure occurrence are identified, this approach can be applied in more data-poor settings to further our current understanding of global submarine slope failure distribution, frequency, and magnitude.