2018 CSDMS meeting-005
Log in (or create account for non-CSDMS members)
Forgot username? Search or email:CSDMSweb@colorado.edu
Browse abstracts
A hydroclimatological approach to predicting regional landslide probability using Landlab
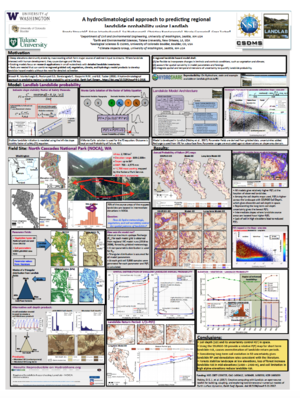
We develop a hydroclimatological approach to modeling regional shallow landslide initiation by integrating spatial and temporal dimensions of parameter uncertainty to estimate an annual probability of landslide initiation based on Monte Carlo simulations. The physically based model couples the infinite-slope stability model with a steady-state subsurface flow representation and operates in a digital elevation model. Spatially distributed gridded data for soil properties and vegetation classification are used for parameter estimation of probability distributions that characterize model input uncertainty. Hydrologic forcing to the model is through annual maximum daily recharge to subsurface flow obtained from a macroscale hydrologic model. We demonstrate the model in a steep mountainous region in northern Washington, USA, over 2700 km2. The influence of soil depth on the probability of landslide initiation is investigated through comparisons among model output produced using three different soil depth scenarios reflecting the uncertainty of soil depth and its potential long-term variability. We found elevation-dependent patterns in probability of landslide initiation that showed the stabilizing effects of forests at low elevations, an increased landslide probability with forest decline at midelevations (1400 to 2400 m), and soil limitation and steep topographic controls at high alpine elevations and in post-glacial landscapes. These dominant controls manifest themselves in a bimodal distribution of spatial annual landslide probability. Model testing with limited observations revealed similarly moderate model confidence for the three hazard maps, suggesting suitable use as relative hazard products. The model is available as a component in Landlab, an open-source, Python-based landscape earth systems modeling environment, and is designed to be easily reproduced utilizing HydroShare cyberinfrastructure.